class: center, middle, inverse, title-slide # How R helped me with time motion analysis ## HAT ### Benjamin Chow --- # Outline - ### 1a) What is time motion study? - ### 1b) What data is needed for time motion study? - ### 2a) Why I should do it in `R`? - ### 2b) How I can I do it in `R`? --- class: inverse, middle, center # What is time motion study? <img src="what is tms.png" widith= "90%"/> --- # Example of "motion" element ### Activities involved in hospital admissions (artificial data) ``` [1] Registration Triage and Assessment Blood test [4] MRI SCAN X-Ray Discuss Results [7] Check-out 7 Levels: Blood test Check-out Discuss Results MRI SCAN ... X-Ray ``` ### There can be different permutations and combinations activities for a work process --- ## Why time motion study? - ### Uncover the sequencing of activities in workflow - ### Identify bottlenecks - ### Identify outliers when compared against a theoretical workflow model - ### Examine the relationship between resource providers --- ## [What did my department use it for?](https://github.com/notast/Time-Motion-Study/blob/main/Conference%20Presentation_Time-motion%20analysis%20for%20productivity.pdf) - ### Examine factors affecting productivity - ### 1. Proportion of inappropriate referrals - ### 2. Total duration for case management (direct and indirect patient activities) --- # Outline - ### ~~1a) What is time motion study?~~ - ### 1b) What data is needed for time motion study? - ### 2a) Why I should do it in `R`? - ### 2b) How I can I do it in `R`? --- count: false # What to collect --- count: false # What to collect ``` # A tibble: 5,442 x 3 time registration_type .order <dttm> <fct> <int> 1 2017-01-02 11:41:53 start 1 2 2017-01-02 11:41:53 start 2 3 2017-01-04 01:34:05 start 3 4 2017-01-04 01:34:04 start 4 5 2017-01-04 16:07:47 start 5 6 2017-01-04 16:07:47 start 6 7 2017-01-05 04:56:11 start 7 8 2017-01-05 04:56:11 start 8 9 2017-01-06 05:58:54 start 9 10 2017-01-06 05:58:54 start 10 # ... with 5,432 more rows ``` --- count: false # What to collect ``` # A tibble: 5,442 x 4 time registration_type .order patient <dttm> <fct> <int> <chr> 1 2017-01-02 11:41:53 start 1 1 2 2017-01-02 11:41:53 start 2 2 3 2017-01-04 01:34:05 start 3 3 4 2017-01-04 01:34:04 start 4 4 5 2017-01-04 16:07:47 start 5 5 6 2017-01-04 16:07:47 start 6 6 7 2017-01-05 04:56:11 start 7 7 8 2017-01-05 04:56:11 start 8 8 9 2017-01-06 05:58:54 start 9 9 10 2017-01-06 05:58:54 start 10 10 # ... with 5,432 more rows ``` --- count: false # What to collect ``` # A tibble: 5,442 x 5 time registration_type .order patient employee <dttm> <fct> <int> <chr> <fct> 1 2017-01-02 11:41:53 start 1 1 r1 2 2017-01-02 11:41:53 start 2 2 r1 3 2017-01-04 01:34:05 start 3 3 r1 4 2017-01-04 01:34:04 start 4 4 r1 5 2017-01-04 16:07:47 start 5 5 r1 6 2017-01-04 16:07:47 start 6 6 r1 7 2017-01-05 04:56:11 start 7 7 r1 8 2017-01-05 04:56:11 start 8 8 r1 9 2017-01-06 05:58:54 start 9 9 r1 10 2017-01-06 05:58:54 start 10 10 r1 # ... with 5,432 more rows ``` <style> .panel1-eventlog-auto { color: black; width: 99%; hight: 32%; float: left; padding-left: 1%; font-size: 80% } .panel2-eventlog-auto { color: black; width: NA%; hight: 32%; float: left; padding-left: 1%; font-size: 80% } .panel3-eventlog-auto { color: black; width: NA%; hight: 33%; float: left; padding-left: 1%; font-size: 80% } </style> --- ### When data is more than _time_ and _motion_, the analysis becomes more of process mining/process analysis ``` Case identifier: patient Activity identifier: handling Resource identifier: employee Activity instance identifier: handling_id Timestamp: time Lifecycle transition: registration_type ``` -- ### For simplicity, we'll call it time motion study --- # Where to collect data for time motion study? - ### Observational Study - ### Self reporting - ### Logs from IT system --- # Outline - ### ~~1a) What is time motion study?~~ - ### ~~1b) What data is needed for time motion study?~~ - ### 2a) Why I should do it in `R`? - ### 2b) How I can I do it in `R`? --- count: false ### 1. Add comments as you formulate your analysis .panel1-why_R_1-auto[ ```r # Boss wants 3 columns from the times tamp # 1 Day of week # 2 Month # 3 Year *patients_df %>% tail(1) %>% select(time) ``` ] .panel2-why_R_1-auto[ ``` # A tibble: 1 x 1 time <dttm> 1 2018-05-03 02:05:09 ``` ] --- count: false ### 1. Add comments as you formulate your analysis .panel1-why_R_1-auto[ ```r # Boss wants 3 columns from the times tamp # 1 Day of week # 2 Month # 3 Year patients_df %>% tail(1) %>% select(time) %>% * mutate( # 1 Day of week * DOW= wday(time, abbr = T), # 2 Month * Month= month(time, abbr = T), # 3 Year * Year= year(time), # keep none of the original columns * .keep="none") ``` ] .panel2-why_R_1-auto[ ``` # A tibble: 1 x 3 DOW Month Year <dbl> <dbl> <dbl> 1 5 5 2018 ``` ] <style> .panel1-why_R_1-auto { color: black; width: 39.2%; hight: 32%; float: left; padding-left: 1%; font-size: 80% } .panel2-why_R_1-auto { color: black; width: 58.8%; hight: 32%; float: left; padding-left: 1%; font-size: 80% } .panel3-why_R_1-auto { color: black; width: NA%; hight: 33%; float: left; padding-left: 1%; font-size: 80% } </style> --- count: false ### 2. Analysis doesn't change if you relocate your columns .panel1-why_R_2-auto[ ```r *patients_df %>% tail(1) ``` ] .panel2-why_R_2-auto[ ``` # A tibble: 1 x 7 handling patient employee handling_id registration_type time <fct> <chr> <fct> <chr> <fct> <dttm> 1 Check-out 494 r7 2721 complete 2018-05-03 02:05:09 # ... with 1 more variable: .order <int> ``` ] --- count: false ### 2. Analysis doesn't change if you relocate your columns .panel1-why_R_2-auto[ ```r patients_df %>% tail(1) %>% * relocate(time, 1) ``` ] .panel2-why_R_2-auto[ ``` # A tibble: 1 x 7 time handling patient employee handling_id registration_type <dttm> <fct> <chr> <fct> <chr> <fct> 1 2018-05-03 02:05:09 Check-out 494 r7 2721 complete # ... with 1 more variable: .order <int> ``` ] --- count: false ### 2. Analysis doesn't change if you relocate your columns .panel1-why_R_2-auto[ ```r patients_df %>% tail(1) %>% relocate(time, 1) %>% * mutate(DOW= wday(time, abbr = T), * Month= month(time, abbr = T), * Year= year(time), * .keep="none") ``` ] .panel2-why_R_2-auto[ ``` # A tibble: 1 x 3 DOW Month Year <dbl> <dbl> <dbl> 1 5 5 2018 ``` ] <style> .panel1-why_R_2-auto { color: black; width: 38.6060606060606%; hight: 32%; float: left; padding-left: 1%; font-size: 80% } .panel2-why_R_2-auto { color: black; width: 59.3939393939394%; hight: 32%; float: left; padding-left: 1%; font-size: 80% } .panel3-why_R_2-auto { color: black; width: NA%; hight: 33%; float: left; padding-left: 1%; font-size: 80% } </style> --- count: false ### 3. Stun your boss's with amazing visualizations 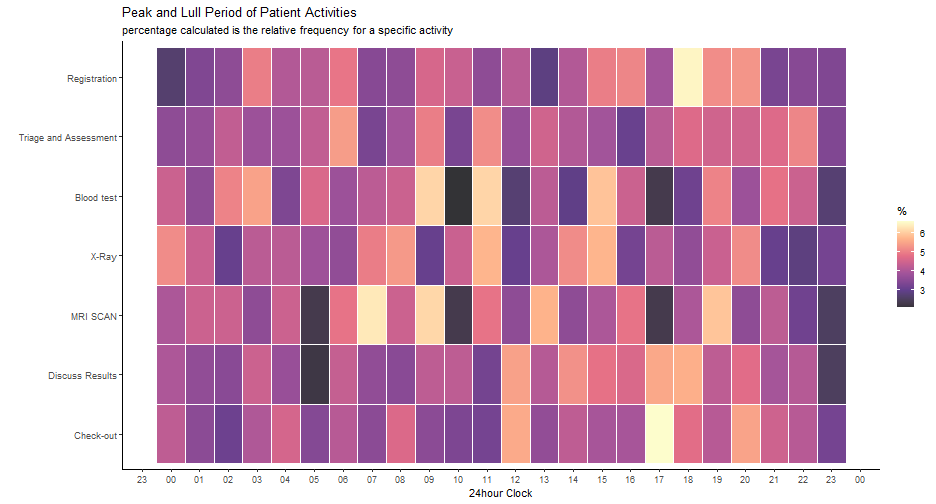<!-- --> --- count: false ### 3. Stun your boss's with amazing visualizations 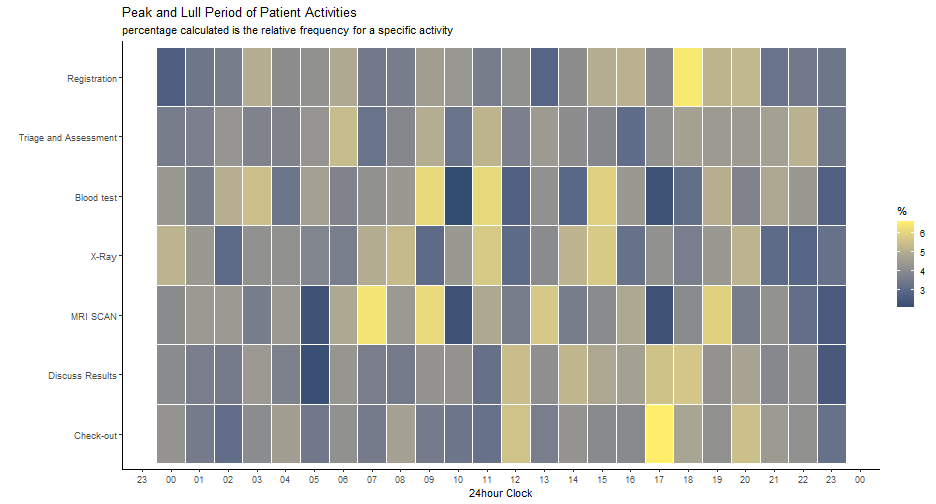<!-- --> --- count: false ### 3. Stun your boss's with amazing visualizations 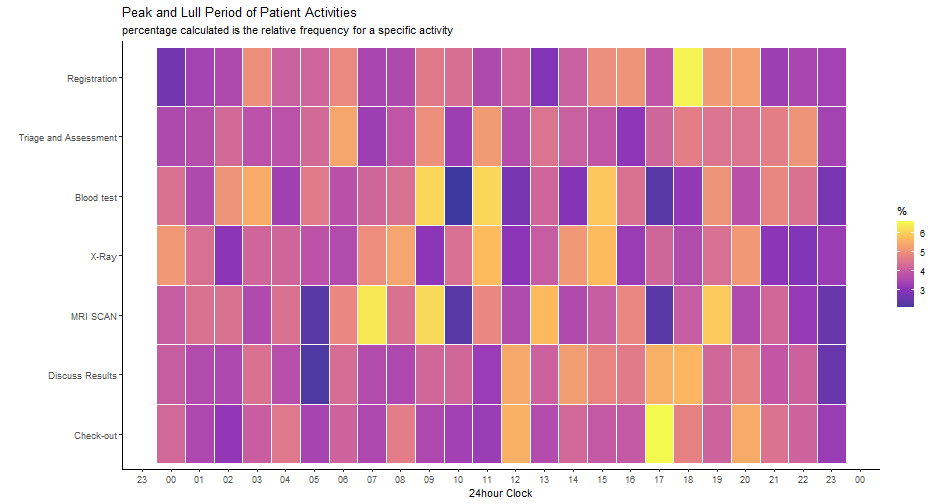<!-- --> <style> .panel1-why_R_3-rotate { color: black; width: 99%; hight: 32%; float: left; padding-left: 1%; font-size: 80% } .panel2-why_R_3-rotate { color: black; width: NA%; hight: 32%; float: left; padding-left: 1%; font-size: 80% } .panel3-why_R_3-rotate { color: black; width: NA%; hight: 33%; float: left; padding-left: 1%; font-size: 80% } </style> --- ## 4a. `R`’s `tidyverse` - ### some languages are harder to learn than others due to their rules - ### programming/scripting languages have rules too - ### `R` has `tidyverse` rules which is beginner friendly - ### `tidyverse` rule 1– verb heavy --- <img src="dplyr_relocate.png" width= "80%"/> ##### Artwork by @allison_horst --- <img src="dplyr_filter.jpg" width= "90%"/> ##### Artwork by @allison_horst --- ## `tidyverse` rule 2– pipeline production `%>%` ```{reval=F} Output <-Input %>% Step 1 %>% Step 2 %>% ... %>% Last step ``` --- ## 4b. `R` packages - ### `R` packages = mobile phone apps - ### `R` has specific packages for time motion analysis that follow `tidyverse` style - ### primary package [`bupaR`](http://bupar.net/). 7 secondary packages --- count: false ### LOS of patients with MRI scan .panel1-why_R_4_MRI-auto[ ```r * patients ``` ] .panel2-why_R_4_MRI-auto[ ``` Log of 5442 events consisting of: 7 traces 500 cases 2721 instances of 7 activities 7 resources Events occurred from 2017-01-02 11:41:53 until 2018-05-05 07:16:02 Variables were mapped as follows: Case identifier: patient Activity identifier: handling Resource identifier: employee Activity instance identifier: handling_id Timestamp: time Lifecycle transition: registration_type # A tibble: 5,442 x 7 handling patient employee handling_id registration_ty~ time <fct> <chr> <fct> <chr> <fct> <dttm> 1 Registrati~ 1 r1 1 start 2017-01-02 11:41:53 2 Registrati~ 2 r1 2 start 2017-01-02 11:41:53 3 Registrati~ 3 r1 3 start 2017-01-04 01:34:05 4 Registrati~ 4 r1 4 start 2017-01-04 01:34:04 5 Registrati~ 5 r1 5 start 2017-01-04 16:07:47 6 Registrati~ 6 r1 6 start 2017-01-04 16:07:47 7 Registrati~ 7 r1 7 start 2017-01-05 04:56:11 8 Registrati~ 8 r1 8 start 2017-01-05 04:56:11 9 Registrati~ 9 r1 9 start 2017-01-06 05:58:54 10 Registrati~ 10 r1 10 start 2017-01-06 05:58:54 # ... with 5,432 more rows, and 1 more variable: .order <int> ``` ] --- count: false ### LOS of patients with MRI scan .panel1-why_R_4_MRI-auto[ ```r patients %>% * filter_activity_presence("MRI SCAN") ``` ] .panel2-why_R_4_MRI-auto[ ``` Log of 2828 events consisting of: 2 traces 236 cases 1414 instances of 6 activities 6 resources Events occurred from 2017-01-02 11:41:53 until 2018-05-04 23:50:05 Variables were mapped as follows: Case identifier: patient Activity identifier: handling Resource identifier: employee Activity instance identifier: handling_id Timestamp: time Lifecycle transition: registration_type # A tibble: 2,828 x 7 handling patient employee handling_id registration_ty~ time <fct> <chr> <fct> <chr> <fct> <dttm> 1 Registrati~ 1 r1 1 start 2017-01-02 11:41:53 2 Registrati~ 3 r1 3 start 2017-01-04 01:34:05 3 Registrati~ 4 r1 4 start 2017-01-04 01:34:04 4 Registrati~ 6 r1 6 start 2017-01-04 16:07:47 5 Registrati~ 7 r1 7 start 2017-01-05 04:56:11 6 Registrati~ 12 r1 12 start 2017-01-08 18:38:19 7 Registrati~ 13 r1 13 start 2017-01-12 09:35:16 8 Registrati~ 15 r1 15 start 2017-01-13 12:09:49 9 Registrati~ 16 r1 16 start 2017-01-13 12:09:49 10 Registrati~ 20 r1 20 start 2017-01-17 22:07:01 # ... with 2,818 more rows, and 1 more variable: .order <int> ``` ] --- count: false ### LOS of patients with MRI scan .panel1-why_R_4_MRI-auto[ ```r patients %>% filter_activity_presence("MRI SCAN") %>% * throughput_time(level="case", * units="days") ``` ] .panel2-why_R_4_MRI-auto[ ``` # Description: case_metric[,2] [236 x 2] patient throughput_time <chr> <dbl> 1 464 23.1 2 370 19.0 3 140 18.9 4 242 18.8 5 93 16.8 6 33 15.6 7 468 14.1 8 374 14.0 9 35 13.8 10 23 13.5 # ... with 226 more rows ``` ] --- count: false ### LOS of patients with MRI scan .panel1-why_R_4_MRI-auto[ ```r patients %>% filter_activity_presence("MRI SCAN") %>% throughput_time(level="case", units="days") %>% * summary() ``` ] .panel2-why_R_4_MRI-auto[ ``` patient throughput_time Length:236 Min. : 1.730 Class :character 1st Qu.: 4.643 Mode :character Median : 6.595 Mean : 7.032 3rd Qu.: 8.750 Max. :23.107 ``` ] <style> .panel1-why_R_4_MRI-auto { color: black; width: 44.1%; hight: 32%; float: left; padding-left: 1%; font-size: 80% } .panel2-why_R_4_MRI-auto { color: black; width: 53.9%; hight: 32%; float: left; padding-left: 1%; font-size: 80% } .panel3-why_R_4_MRI-auto { color: black; width: NA%; hight: 33%; float: left; padding-left: 1%; font-size: 80% } </style> --- count: false ### LOS of patients without MRI scan .panel1-why_R_4_noMRI-auto[ ```r *patients ``` ] .panel2-why_R_4_noMRI-auto[ ``` Log of 5442 events consisting of: 7 traces 500 cases 2721 instances of 7 activities 7 resources Events occurred from 2017-01-02 11:41:53 until 2018-05-05 07:16:02 Variables were mapped as follows: Case identifier: patient Activity identifier: handling Resource identifier: employee Activity instance identifier: handling_id Timestamp: time Lifecycle transition: registration_type # A tibble: 5,442 x 7 handling patient employee handling_id registration_ty~ time <fct> <chr> <fct> <chr> <fct> <dttm> 1 Registrati~ 1 r1 1 start 2017-01-02 11:41:53 2 Registrati~ 2 r1 2 start 2017-01-02 11:41:53 3 Registrati~ 3 r1 3 start 2017-01-04 01:34:05 4 Registrati~ 4 r1 4 start 2017-01-04 01:34:04 5 Registrati~ 5 r1 5 start 2017-01-04 16:07:47 6 Registrati~ 6 r1 6 start 2017-01-04 16:07:47 7 Registrati~ 7 r1 7 start 2017-01-05 04:56:11 8 Registrati~ 8 r1 8 start 2017-01-05 04:56:11 9 Registrati~ 9 r1 9 start 2017-01-06 05:58:54 10 Registrati~ 10 r1 10 start 2017-01-06 05:58:54 # ... with 5,432 more rows, and 1 more variable: .order <int> ``` ] --- count: false ### LOS of patients without MRI scan .panel1-why_R_4_noMRI-auto[ ```r patients %>% * filter_activity_presence("MRI SCAN", * method="none") ``` ] .panel2-why_R_4_noMRI-auto[ ``` Log of 2614 events consisting of: 5 traces 264 cases 1307 instances of 6 activities 6 resources Events occurred from 2017-01-02 11:41:53 until 2018-05-05 07:16:02 Variables were mapped as follows: Case identifier: patient Activity identifier: handling Resource identifier: employee Activity instance identifier: handling_id Timestamp: time Lifecycle transition: registration_type # A tibble: 2,614 x 7 handling patient employee handling_id registration_ty~ time <fct> <chr> <fct> <chr> <fct> <dttm> 1 Registrati~ 2 r1 2 start 2017-01-02 11:41:53 2 Registrati~ 5 r1 5 start 2017-01-04 16:07:47 3 Registrati~ 8 r1 8 start 2017-01-05 04:56:11 4 Registrati~ 9 r1 9 start 2017-01-06 05:58:54 5 Registrati~ 10 r1 10 start 2017-01-06 05:58:54 6 Registrati~ 11 r1 11 start 2017-01-08 18:38:19 7 Registrati~ 14 r1 14 start 2017-01-12 09:35:16 8 Registrati~ 17 r1 17 start 2017-01-16 00:42:38 9 Registrati~ 18 r1 18 start 2017-01-16 00:42:38 10 Registrati~ 19 r1 19 start 2017-01-17 22:07:01 # ... with 2,604 more rows, and 1 more variable: .order <int> ``` ] --- count: false ### LOS of patients without MRI scan .panel1-why_R_4_noMRI-auto[ ```r patients %>% filter_activity_presence("MRI SCAN", method="none") %>% * throughput_time(level="case", * units="days") ``` ] .panel2-why_R_4_noMRI-auto[ ``` # Description: case_metric[,2] [264 x 2] patient throughput_time <chr> <dbl> 1 466 19.1 2 383 15.4 3 467 15.0 4 145 14.3 5 316 13.9 6 98 13.8 7 71 13.7 8 276 13.4 9 40 12.9 10 74 12.7 # ... with 254 more rows ``` ] --- count: false ### LOS of patients without MRI scan .panel1-why_R_4_noMRI-auto[ ```r patients %>% filter_activity_presence("MRI SCAN", method="none") %>% throughput_time(level="case", units="days") %>% * summary() ``` ] .panel2-why_R_4_noMRI-auto[ ``` patient throughput_time Length:264 Min. : 1.496 Class :character 1st Qu.: 4.171 Mode :character Median : 5.612 Mean : 6.359 3rd Qu.: 8.425 Max. :19.126 ``` ] <style> .panel1-why_R_4_noMRI-auto { color: black; width: 44.1%; hight: 32%; float: left; padding-left: 1%; font-size: 80% } .panel2-why_R_4_noMRI-auto { color: black; width: 53.9%; hight: 32%; float: left; padding-left: 1%; font-size: 80% } .panel3-why_R_4_noMRI-auto { color: black; width: NA%; hight: 33%; float: left; padding-left: 1%; font-size: 80% } </style> --- # 5. One stop shop <img src="rmarkdown_rockstar.png" width= "60%"/> ##### Artwork by @allison_horst --- <img src="rmarkdown_wizards.png" width= "80%"/> ##### Artwork by @allison_horst --- # 6. As text/ code/ comments of your analysis are in one place, it is reproducible. <img src="reproducibility_court.png" width= "60%"/> ##### Artwork by @allison_horst --- # Outline - ### ~~1a) What is time motion study?~~ - ### ~~1b) What data is needed for time motion study?~~ - ### 2a) ~~Why I should do it in `R`?~~ - ### 2b) How I can I do it in `R`? --- ### Boss _"I heard you attended HAT."_ -- ### You _"Yeah, why?"_ -- ### Boss _"There are so many patients coming in at different times and doing different activities. Quite hard to visualize their movements."_ -- ### Boss _"Can you use your HAT skills to create a flow map from the time patients were admitted to discharge?"_ --- ### Getting you comfortable to use `bupaR` in `R` <img src="r_first_then.png" width= "90%"/> ##### Artwork by @allison_horst --- # setup ```r # the packages library(tidyverse) library(bupaR) # primary library(processanimateR) # secondary package which is not automatically loaded with bupaR ``` -- ```r # import data dataset<- read_csv("file name.csv") # convert to format recognized by bupaR dataset_bupaR_format<- dataset %>% eventlog( case_id = "patient", activity_id = "activity", activity_instance_id = "activity_instance", lifecycle_id = "status", timestamp = "timestamp", resource_id = "resource" ) ``` --- ``` # A tibble: 5,442 x 7 handling patient employee handling_id registration_ty~ time <fct> <chr> <fct> <chr> <fct> <dttm> 1 Registrati~ 1 r1 1 start 2017-01-02 11:41:53 2 Registrati~ 2 r1 2 start 2017-01-02 11:41:53 3 Registrati~ 3 r1 3 start 2017-01-04 01:34:05 4 Registrati~ 4 r1 4 start 2017-01-04 01:34:04 5 Registrati~ 5 r1 5 start 2017-01-04 16:07:47 6 Registrati~ 6 r1 6 start 2017-01-04 16:07:47 7 Registrati~ 7 r1 7 start 2017-01-05 04:56:11 8 Registrati~ 8 r1 8 start 2017-01-05 04:56:11 9 Registrati~ 9 r1 9 start 2017-01-06 05:58:54 10 Registrati~ 10 r1 10 start 2017-01-06 05:58:54 # ... with 5,432 more rows, and 1 more variable: .order <int> ``` --- # Create a flow map ### Journey of 500 patients across a fixed time period ```r patients %>% process_map() ```
--- # Animate it ### The journey of the patients at specific time points ```r patients %>% animate_process() ```
--- count: false ### It’s okay if you don’t know https://bupaverse.github.io/processanimateR/reference/animate_process.html .panel1-animate_duration-replace[ ```r patients %>% * animate_process(duration=60) ``` ] .panel2-animate_duration-replace[
] --- count: false ### It’s okay if you don’t know https://bupaverse.github.io/processanimateR/reference/animate_process.html .panel1-animate_duration-replace[ ```r patients %>% * animate_process(duration=30) ``` ] .panel2-animate_duration-replace[
] <style> .panel1-animate_duration-replace { color: black; width: 99%; hight: 32%; float: top; padding-left: 1%; font-size: 80% } .panel2-animate_duration-replace { color: black; width: NA%; hight: 32%; float: top; padding-left: 1%; font-size: 80% } .panel3-animate_duration-replace { color: black; width: NA%; hight: 33%; float: top; padding-left: 1%; font-size: 80% } </style> --- - ### Boss _"I heard you attended HAT."_ - ### You _"Yeah, why?"_ - ### Boss _"There are so many patients coming in at different times and doing different activities. Quite hard to visualize their movements."_ - ### Boss _"Can you use your HAT skills to create a flow map from the time patients were admitted to discharge?"_ -- - ### You _"Here you go boss."_ --- ```r animate_process(patients, #slow down, easier to spot bottleneck duration=200, # a colour for each pt mapping = token_aes(color = token_scale("patient",scale = "ordinal", range = RColorBrewer::brewer.pal(12, "Paired")))) ```
--- # Any Questions <img src="foreveR.jfif" width= "70%"/> ##### Artwork by @allison_horst